IEEETGRS2024 – Stereollax Net: Stereo Parallax Based Deep Learning Network For Building Height Estimation
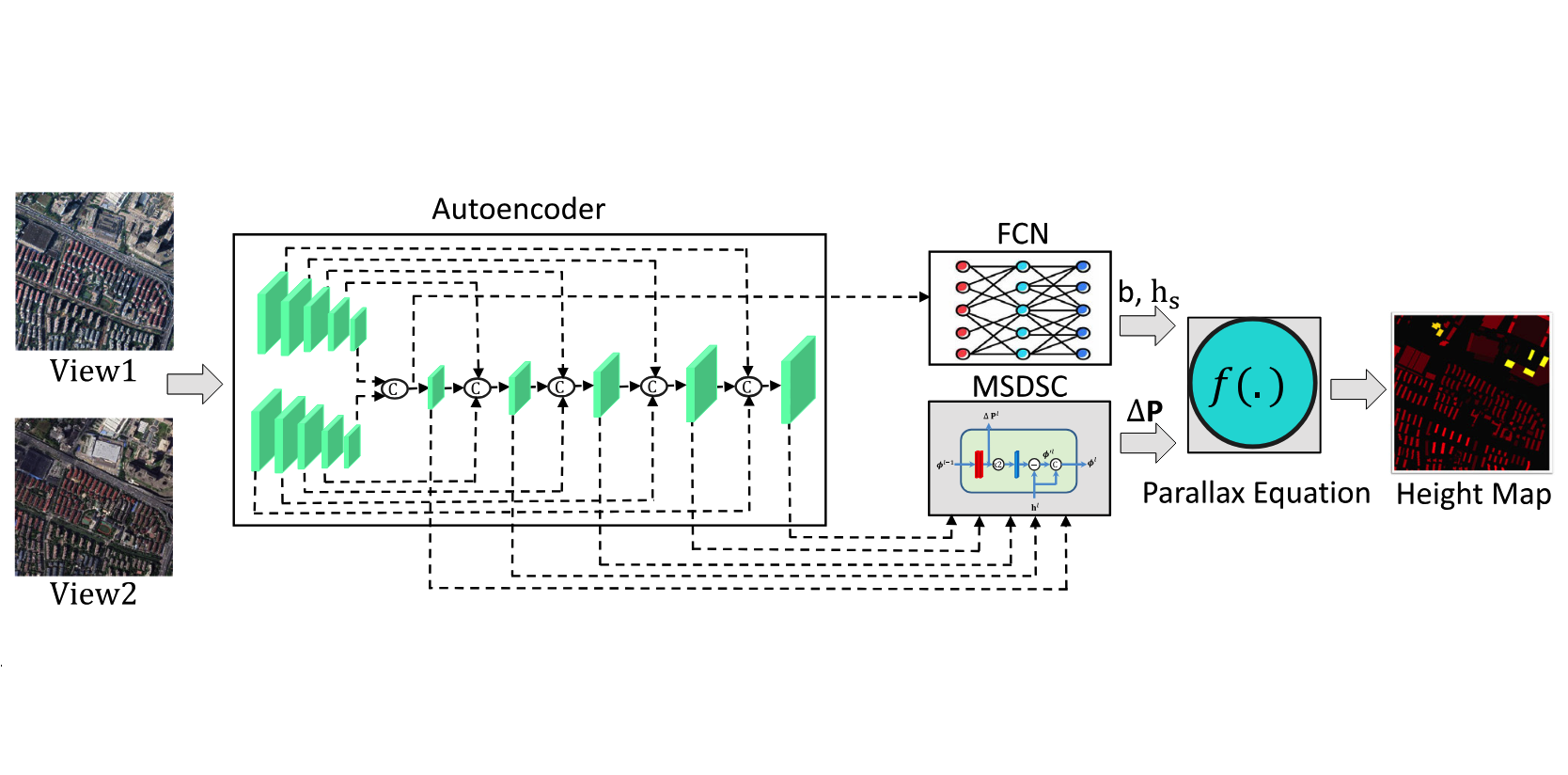
Sana Jabbar and Murtaza Taj
Abstract:
Accurate estimation of building heights is crucial for effective urban planning and resource management as it provides essential geometric information about the urban landscape. Many end-to-end deep learning-based networks have been proposed for image-to-height mapping using high-resolution nonoptical and optical remote sensing imagery. In this study, we develop a novel deep-learning architecture that incorporates a stereo parallax-based mathematical formulation for building height estimation. We estimate stereo formulation parameters include differential parallax ( ΔP ) image, average photo-base, and satellite height). The final height map is computed by utilizing these parameters in the stereo parallax equation, thus combining closed-form solutions within the learning paradigm. Moreover, to improve the estimation of ΔP , we also introduce a multiscale differential shortcut connection (MSDSC) module. The MSDSC module integrates high-frequency components into lower resolution baseline decoder features while converting them into high-resolution decoder features. To establish the efficacy of our proposed stereo parallax-based deep learning network (Stereollax Net), we train and evaluate our method on densely populated cities of China (42-Cities dataset) and on the IEEE Data Fusion Contest 2018 (DFC2018) dataset. Our proposed Stereollax Net is trained only with RGB imagery and compared with the state-of-the-art (SOTA) methods that utilize both panchromatic and multispectral (RGB and near-infrared) satellite imagery. The qualitative and quantitative results demonstrate that our Stereollax Net surpasses existing SOTA algorithms, achieving superior performance with fewer data and training parameters by a considerable margin. The code will be made publicly available via the GitHub repository.
Text Reference:
S. Jabbar and M. Taj, "Stereollax Net: Stereo Parallax Based Deep Learning Network For Building Height Estimation," in IEEE Transactions on Geoscience and Remote Sensing, 2024
Bibtex Reference:
@ARTICLE{10497089, author={Jabbar, Sana and Taj, Murtaza}, journal={IEEE Transactions on Geoscience and Remote Sensing}, title={Stereollax Net: Stereo Parallax-Based Deep Learning Network for Building Height Estimation}, year={2024}, volume={62}, number={}, pages={1-12}, keywords={Buildings;Estimation;Optical imaging;Optical sensors;Decoding;Urban areas;Satellites;Autoencoder;building height;fully connected network (FCN);optical imagery;stereo parallax}, doi={10.1109/TGRS.2024.3387476}}