ICASSP 2019- Point Cloud Segmentation Using Hierarchical Tree for Architectural Models
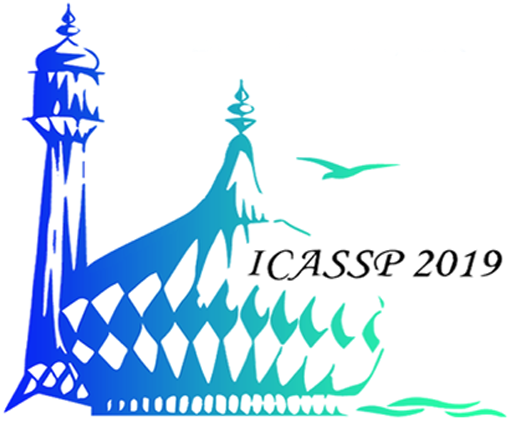
Omair Hassaan, Abeera Shamail, Zain Butt, Murtaza Taj
International Conference on Acoustics, Speech and Signal Processing (ICASSP), Brighton, UK, May 12-17, 2019
Abstract
Over the past few years, gathering massive volume of 3D data has become straightforward due to the proliferation of laser scanners and acquisition devices. Segmentation of such large data into meaningful segments, however, remains a challenge. Raw scans usually have missing data and varying density. In this work, we present a simple yet effective method to semantically decompose and reconstruct 3D models from point clouds. Using a hierarchical tree approach, we segment and reconstruct planar as well as non-planar scenes in an outdoor environment. This tree uses an exclusive energy function and a 3D convolutional neural network, HollowNets, to classify the segments. We test the efficacy of our proposed approach on a variety of complex real and synthetic data samples, obtaining an improvement of 7.9% in mean IOU over the state of the art approaches.
Text Reference:
Omair Hassaan, Abeera Shamail, Zain Butt, Murtaza Taj, "Point Cloud Segmentation Using Hierarchical Tree for Architectural Models", International Conference on Acoustics, Speech and Signal Processing (ICASSP), Brighton, UK, May 12-17, 2019
Bibtex Reference:
@inproceedings{Hassaan3DPointICASSP2019, author = "Omair Hassaan and Abeera Shamail and Zain Butt and Murtaza Taj", title = "Point Cloud Segmentation Using Hierarchical Tree for Architectural Models", booktitle = "International Conference on Acoustics, Speech and Signal Processing (ICASSP)", month = "May ", year = "2019", }