ICASSP 2019 – Adaptively Weighted Multi-Task Learning Using Inverse Validation Loss
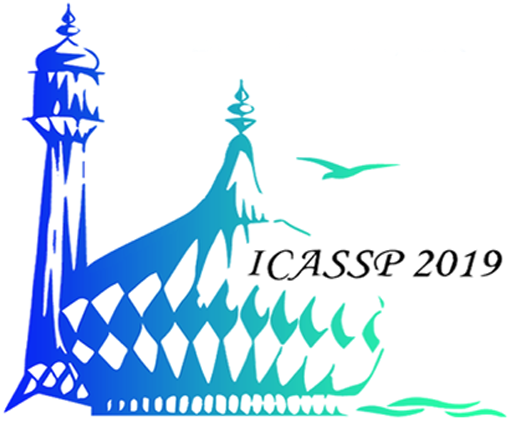
Waseem Abbas and Murtaza Taj
International Conference on Acoustics, Speech and Signal Processing (ICASSP), Brighton, UK, May 12-17, 2019
Abstract
Multi-task learning aims to enhance the performance of a model by inductive transfer of information among tasks. However, joint optimization of multiple tasks is challenging due to unbalanced data ranges and variations in task difficulties which can cause the model to converge only for a single task which has large values. To address these problems, we propose a novel weighting scheme based on validation loss. The proposed weighted scheme is evaluated on three datasets, including publicly available Comma.ai and Udacity benchmark dataset and GTA-V dataset. Our experiments demonstrate the superior performance of the proposed approach compared to the current state-of-the-art methods.
Text Reference:
Waseem Abbas and Murtaza Taj, "Adaptively Weighted Multi-Task Learning Using Inverse Validation Loss", International Conference on Acoustics, Speech and Signal Processing (ICASSP), Brighton, UK, May 12-17, 2019
Bibtex Reference:
@inproceedings{WaseemSDCICASSP2019, author = "Waseem Abbas and Murtaza Taj", title = "Adaptively Weighted Multi-Task Learning Using Inverse Validation Loss", booktitle = "International Conference on Acoustics, Speech and Signal Processing (ICASSP)", month = "May ", year = "2019", }